Communications coordinator Catherine Meister attended the inaugural Australian AI Safety Forum (November 7 – 8, 2024), at the University of Sydney. The Sydney Mathematical Research Institute was one of the sponsors of the forum.
When it comes to the defining technological developments over the last decades, what springs to mind? Perhaps the rise of smart phones? If you have asked GPT to write a wedding speech, a cover letter, or used an image generator such as Midjourney, there is a good chance your answer will be advances in artificial intelligence (AI).
While high-profile AI advances have captured our attention in recent years, the concept of AI was first explored in literature 70 years ago. Cryptologist Alan Turing is famous for his work cracking the German WWII ciphers, as well as his contributions to fundamental computer science research. He was also one of the first scientists to explore what a ‘thinking machine’ could look like, devising the first benchmark for AI: the Turing test.
Today’s large language models (LLMs) convincingly pass the Turing test. Regulation and responsible use of these technologies is now a topic of interest to the general public, as well as researchers and industry. In November 2024, the inaugural Australian AI Safety Forum was held at the Sydney Knowledge Hub. The purpose of the forum was to bring together diverse parties: researchers, government, industry, policy and lawmakers to discuss what safe and responsible use of AI might look like in Australia.
The current state of AI safety
Currently, we have a window of opportunity to regulate use of AI. Risks of inappropriate use of AI range from its use in warfare to production of misinformation and conducting scams. However, despite clear dangers, even AI researchers can’t agree on whether AI provides an existential threat to humanity. Organisations are beginning to provide guidelines to promote the safe and responsible use of AI. These include the AI Seoul Summit 2024, and the Bletchley Declaration of 2023, established in Bletchley Park, with its historic links to Turing and computer science.
AI systems have a range of abilities, from models with specific uses (LLMs, image or video generators, AlphaGo, AlphaFold) to general purpose artificial intelligence (GPAI). GPAI’s attention can be turned to a wide range of problems or outputs and may demonstrate complex reasoning processes in line with average human intelligence. Models that perform above the capacity of an average human are termed artificial super intelligence. Clearly, computational capacity exceeding that of an average human is a benchmark, although it remains difficult to develop tools to assess abilities to reason.
The dangers of AI include its scalability
Increases in compute, training time and data are all required to scale up AI. Increases in all three factors lead to improved capabilities, increased speed, and efficiency in computation. These gains correlate directly to improved output in terms of system intelligence. In this situation, money can buy computational power and, in turn, intelligence! Naturally, this could have devastating societal impacts. Some AI experts believe that a predicted 10,000 fold improvement in computational power by 2032 may make GPAI achievable. These developments may outpace both regulation and human abilities to reason.
So what does machine learning look like?
Most readers will be familiar with AI’s ability to learn Go or chess to a human or above human standard. How is it that GPT can write lucid sentences in response to a prompt? LLMs such as GPT scour their training data to become proficient at predicting the next token in a sequence of tokens. The ‘intelligence’ to perform these tasks is developed by a framework known as a neural net, which has layers or loops of feedback which allow the system to evaluate and improve its output. This process is somewhat analogous to human learning, in that poor outcomes can lead to eventual improvements.
While there are similarities, there are also vital differences in the way machines ‘learn’ how to solve problems. Systems such as GPT have no earthly context for their intelligence and learning – their experience of the world is restricted to information contained in the training data, which is reduced to a sequence of tokens. This is very different from the human experience, which is rich in qualia! Abstraction comes naturally to humans, even with smaller training sets.
Why is AI so interesting? And why shouldn’t we just stop all research into AI?
In Computing Intelligence and Machinery, Turing highlighted five key areas of interest for AI, one of which was mathematical research. Today, tasks involving high levels of mathematical reasoning pose a significant challenge to AI systems. Suitable training data are limited, so logic and reasoning are far more challenging for AI than pattern recognition (LLMs). The application of AI to these complex problems is therefore a means to access solutions to open problems in mathematics, as well as a philosophical exercise. Exploring whether reasoning processes are uniquely human allows us to explore the limits of both artificial and human intelligence.
Exploring machine learning for mathematics
Alongside François Charton, Jordan S. Ellenberg, and Adam Zsolt Wagner, SMRI Director Geordie Williamson recently published findings that training neural networks was found to be a flexible and effective method to discover interesting constructions in mathematics. For Geordie, using AI systems is another tool we can use to investigate open problems in mathematics. He prefers to think of AI as just one of many axes of intelligence that form the toolkit of a working mathematician.
To regulate or not to regulate?
AI might be helpful in scientific areas that require dredging complex data sets for useful information, with implications for areas such as climate science, government regulation or medicine. Too much AI regulation may stifle discovery of solutions to complex problems facing humanity today, however not enough regulation leaves us at risk of other problems, with severe consequences for knowledge work.
At the Australian AI Safety Forum, there was much discussion of Australia’s geopolitical role in AI safety. As a middle power, Australia could lead by example on AI safety and may act as a broker between larger regional powers such as the US and China. Recently, the Department of Industry, Science and Resources published 10 voluntary AI safety guardrails, Australia’s first foray into AI regulation. Establishing a centre for Australian AI safety may be the next step.
Why is maths more important than ever?
In a post-AI world, individuals need to rely on their knowledge of the world to evaluate the credibility of information generated by systems such as LLMs. It’s harder than ever to come across good quality information. While LLMs can pass the Turing Test and may quickly answer a set of homework questions, critical thinking is needed to validate the accuracy and reliability of the output of these tools. As physicist Richard Feynman noted, ‘Knowledge isn’t free. You have to pay attention.’ When used responsibly, AI is a useful tool to supplement other problem-solving skills. Calls for the regulation and responsible use of AI are incredibly important, but the rise in AI also highlights the importance of mathematics in societies today, as the fundamental tool to understand the algorithms and frameworks for developing the models shaping our world.
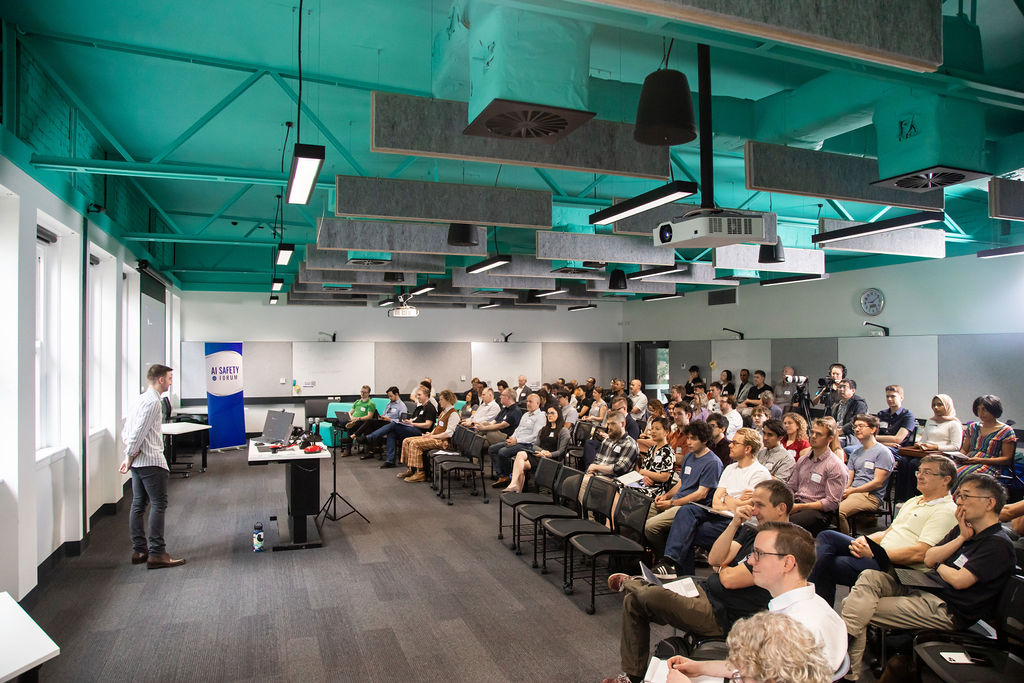
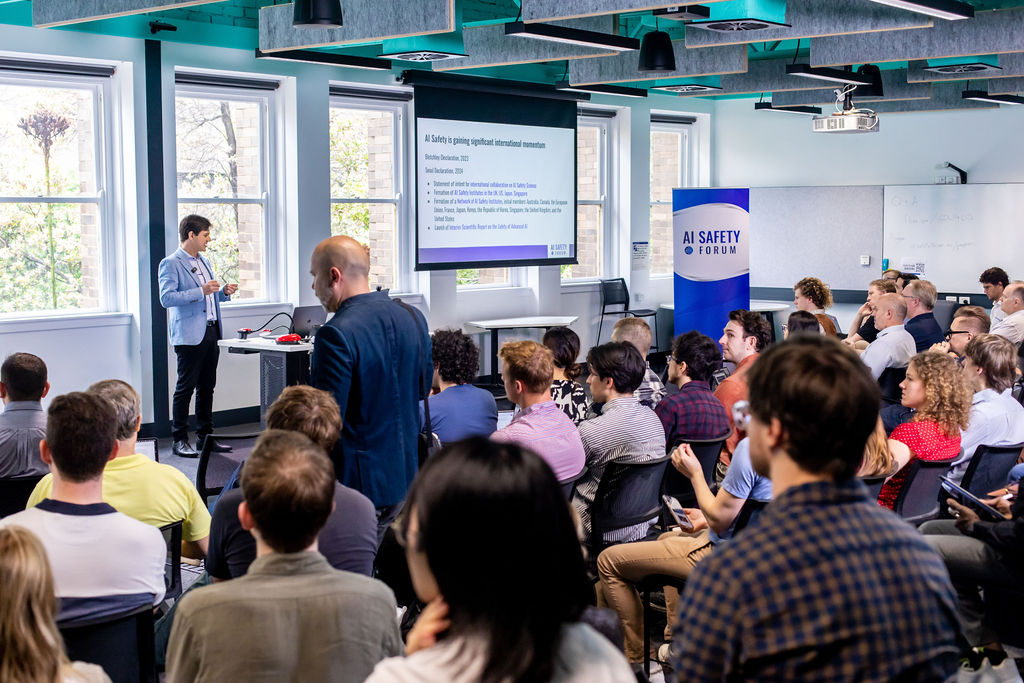
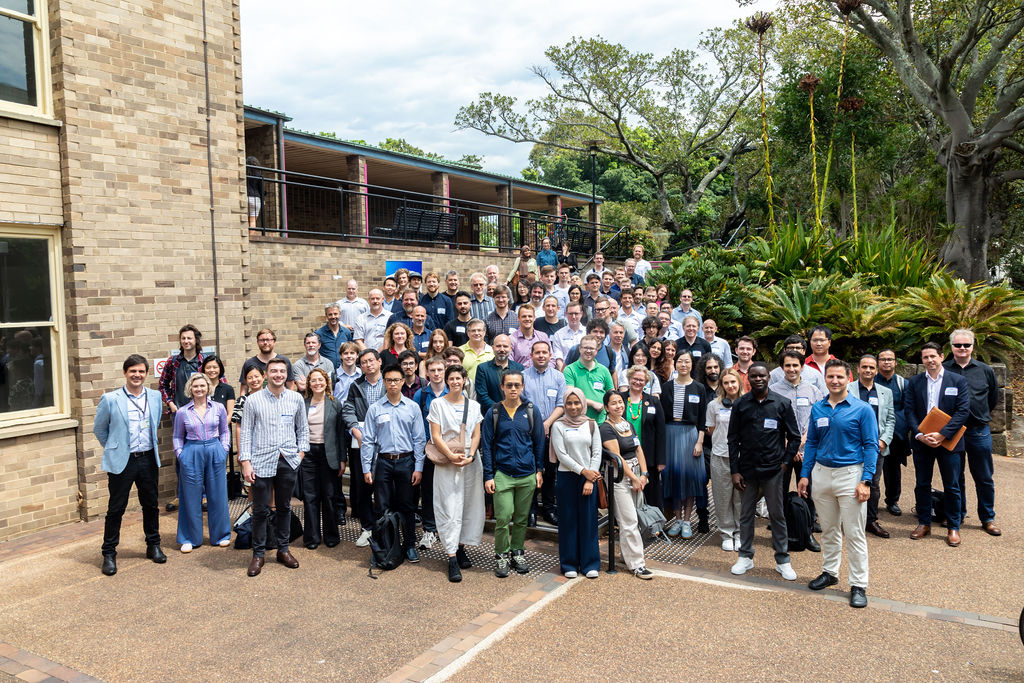
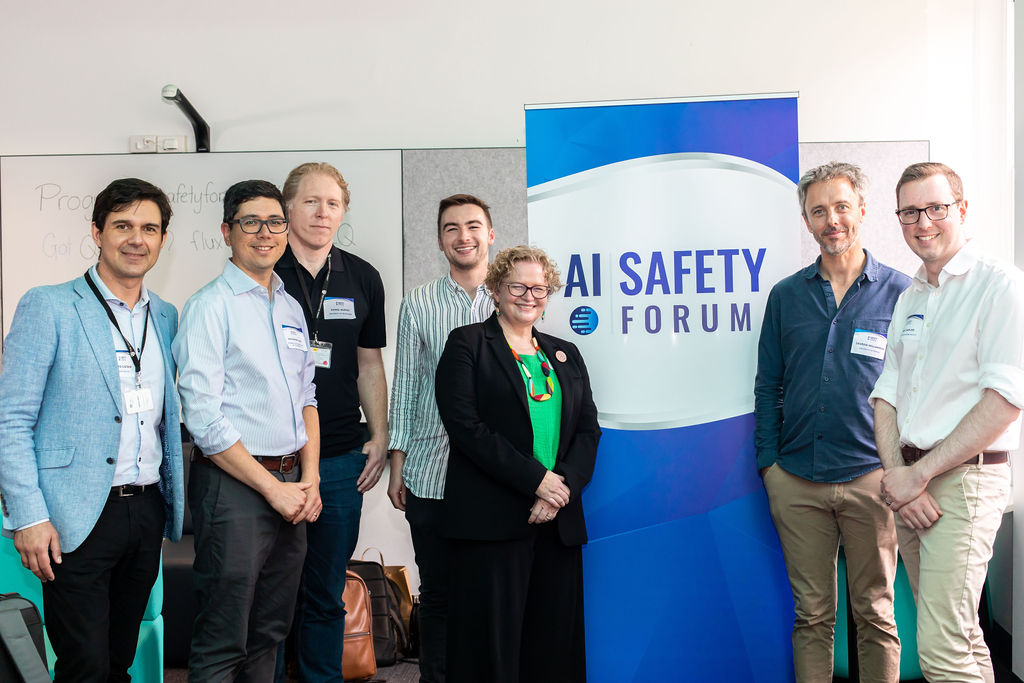